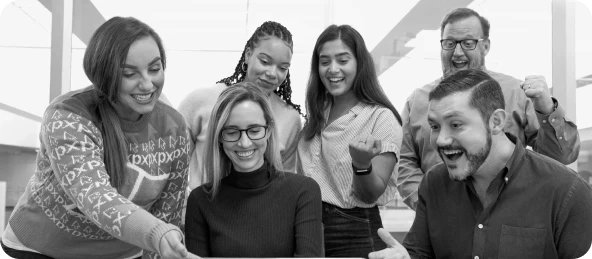
Cost Analysis in AI Services: Breaking Down Expenses and Maximizing ROI
An in-depth look at the true costs behind AI services. We explore why cost analysis is crucial, identify key expense components (data, cloud infrastructure, talent, etc.), introduce methods like TCO, CBA, and ROI for evaluating AI investments, and share strategies to optimize costs while maximizing return on investment.
Introduction
Implementing artificial intelligence (AI) can greatly enhance efficiency and decision-making in businesses. However, these benefits come with significant costs that need careful analysis WALTURN.COM.
Without understanding the expenses behind AI services, projects risk running over budget or failing to deliver expected value. In fact, at scale AI costs can grow exponentially, making cost analysis crucial for informed decision-making and ensuring a positive return on investment MOESIF.COM.
For business leaders, analyzing costs upfront and throughout an AI initiative helps in budgeting effectively, managing resources, and maximizing the value derived from AI.
Key Cost Components
Cost drivers for AI services span several areas. Understanding these key cost components is the first step in effective cost analysis. Below we break down major factors – from acquiring data to maintaining AI systems – that contribute to the overall expenditure of AI projects.
Data Acquisition and Management
Quality data is the fuel for AI, and obtaining it often incurs substantial expenses. Organizations may need to collect, purchase, or label large datasets, and ensure that the data meet quality and privacy standards. The cost of acquiring, storing, and managing data can be significant, especially in regulated industries that require compliance measures (e.g., anonymization for healthcare data) WALTURN.COM.
Ongoing costs include cloud storage fees for massive datasets, data cleaning and integration into existing systems, and implementing security to protect sensitive information. All these efforts are necessary investments to provide the reliable data foundation that AI models require.
Cloud Computing and Infrastructure
AI workloads demand heavy computing power – typically utilizing GPUs, TPUs, or other specialized hardware – which translates to notable infrastructure costs. Businesses must decide between cloud-based computing and on-premises infrastructure for their AI services. Cloud platforms (like AWS, Google Cloud, or Azure) offer scalable, pay-as-you-go resources, but usage costs can spike unpredictably as demand grows WALTURN.COM.
On the other hand, building in-house infrastructure means high upfront capital expenditure on servers and equipment, plus ongoing expenses for power, cooling, and hardware maintenance. While on-premise hardware can be cost-effective in the long run for large consistent workloads, it also requires hiring specialized IT staff to manage it. In summary, AI computing infrastructure – whether rented in the cloud or owned outright – is a major budget item. Companies need to weigh the stable but high initial costs of on-premises systems against the flexible but variable costs of cloud computing WALTURN.COM.
Talent and Expertise
Skilled people are often the most expensive component of AI services. Developing and deploying AI solutions requires AI researchers, data scientists, machine learning engineers, and other specialists – roles that are in high demand and command premium salaries. Hiring experienced AI talent can mean paying six-figure salaries (ranging roughly from $100k to $300k annually) for each expert WALTURN.COM.
In addition to salaries, there are costs for benefits, continuous training, or certifications to keep skills up to date. Some companies mitigate talent costs by upskilling existing staff or outsourcing AI development to external agencies, but these approaches have trade-offs in capability or control. Whether in-house or contracted, investment in talent is critical – AI initiatives will stall without the right expertise, and acquiring that expertise significantly impacts the overall cost of AI services.
Ongoing Maintenance
AI services are not a one-time expense – after deployment, they require continuous maintenance and updates. Models can degrade in accuracy over time as data patterns change, a phenomenon known as model drift. Thus, businesses must budget for regular monitoring, retraining, and tuning of AI models to keep them performing well. There are also recurring costs for software licenses, cloud service subscriptions, and support. As security threats evolve, AI systems need security updates and data privacy audits to remain compliant.
In short, ongoing maintenance is an essential cost to plan for. AI systems “degrade over time, necessitating continuous retraining, monitoring, and security updates to maintain performance” WALTURN.COM. Overlooking maintenance can lead to reduced AI effectiveness and even system failures, so prudent cost analysis treats post-deployment expenses as equally important as initial development costs.